... ...
# LSTMs for Human Activity Recognition
Human activity recognition using smartphones dataset and an LSTM RNN. Classifying the type of movement amongst six categories:
- WALKING,
- WALKING_UPSTAIRS,
- WALKING_DOWNSTAIRS,
- SITTING,
- STANDING,
- LAYING.
Compared to a classical approach, using a Recurrent Neural Networks (RNN) with Long Short-Term Memory cells (LSTMs) require no or almost no feature engineering. Data can be fed directly into the neural network who acts like a black box, modeling the problem correctly. Other research on the activity recognition dataset used mostly use a big amount of feature engineering, which is rather a signal processing approach combined with classical data science techniques. The approach here is rather very simple in terms of how much did the data was preprocessed.
## Video dataset overview
Follow this link to see a video of the 6 activities recorded in the experiment with one of the participants:
[Watch video]
## Details about input data
I will be using an LSTM on the data to learn (as a cellphone attached on the waist) to recognise the type of activity that the user is doing. The dataset's description goes like this:
> The sensor signals (accelerometer and gyroscope) were pre-processed by applying noise filters and then sampled in fixed-width sliding windows of 2.56 sec and 50% overlap (128 readings/window). The sensor acceleration signal, which has gravitational and body motion components, was separated using a Butterworth low-pass filter into body acceleration and gravity. The gravitational force is assumed to have only low frequency components, therefore a filter with 0.3 Hz cutoff frequency was used.
That said, I will use the almost raw data: only the gravity effect has been filtered out of the accelerometer as a preprocessing step for another 3D feature as an input to help learning.
## What is an RNN?
As explained in [this article](http://karpathy.github.io/2015/05/21/rnn-effectiveness/), an RNN takes many input vectors to process them and output other vectors. It can be roughly pictured like in the image below, imagining each rectangle has a vectorial depth and other special hidden quirks in the image below. **In our case, the "many to one" architecture is used**: we accept time series of feature vectors (one vector per time step) to convert them to a probability vector at the output for classification. Note that a "one to one" architecture would be a standard feedforward neural network.
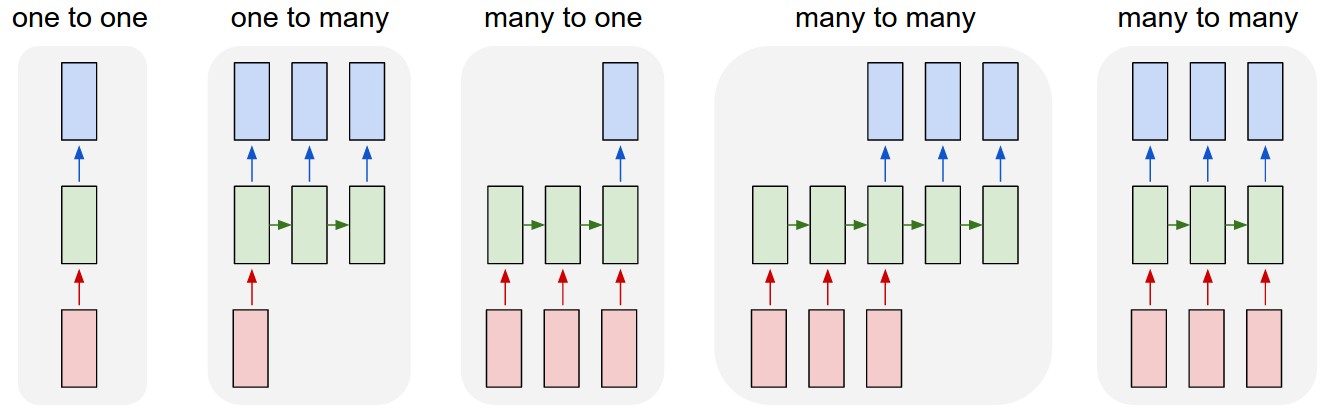
An LSTM is an improved RNN. It is more complex, but easier to train, avoiding what is called the vanishing gradient problem.
## Results
Scroll on! Nice visuals awaits.
```python
# All Includes
import numpy as np
import matplotlib
import matplotlib.pyplot as plt
import tensorflow as tf # Version 1.0.0 (some previous versions are used in past commits)
from sklearn import metrics
import os
```
```python
# Useful Constants
# Those are separate normalised input features for the neural network
INPUT_SIGNAL_TYPES = [
"body_acc_x_",
"body_acc_y_",
"body_acc_z_",
"body_gyro_x_",
"body_gyro_y_",
"body_gyro_z_",
"total_acc_x_",
"total_acc_y_",
"total_acc_z_"
]
# Output classes to learn how to classify
LABELS = [
"WALKING",
"WALKING_UPSTAIRS",
"WALKING_DOWNSTAIRS",
"SITTING",
"STANDING",
"LAYING"
]
```
## Let's start by downloading the data:
```python
# Note: Linux bash commands start with a "!" inside those "ipython notebook" cells
DATA_PATH = "data/"
!pwd && ls
os.chdir(DATA_PATH)
!pwd && ls
!python download_dataset.py
!pwd && ls
os.chdir("..")
!pwd && ls
DATASET_PATH = DATA_PATH + "UCI HAR Dataset/"
print("\n" + "Dataset is now located at: " + DATASET_PATH)
```
/home/ubuntu/pynb/LSTM-Human-Activity-Recognition
data LSTM_files LSTM_OLD.ipynb README.md
LICENSE LSTM.ipynb lstm.py screenlog.0
/home/ubuntu/pynb/LSTM-Human-Activity-Recognition/data
download_dataset.py source.txt
Downloading...
--2017-05-24 01:49:53-- https://archive.ics.uci.edu/ml/machine-learning-databases/00240/UCI%20HAR%20Dataset.zip
Resolving archive.ics.uci.edu (archive.ics.uci.edu)... 128.195.10.249
Connecting to archive.ics.uci.edu (archive.ics.uci.edu)|128.195.10.249|:443... connected.
HTTP request sent, awaiting response... 200 OK
Length: 60999314 (58M) [application/zip]
Saving to: ‘UCI HAR Dataset.zip’
100%[======================================>] 60,999,314 1.69MB/s in 38s
2017-05-24 01:50:31 (1.55 MB/s) - ‘UCI HAR Dataset.zip’ saved [60999314/60999314]
Downloading done.
Extracting...
Extracting successfully done to /home/ubuntu/pynb/LSTM-Human-Activity-Recognition/data/UCI HAR Dataset.
/home/ubuntu/pynb/LSTM-Human-Activity-Recognition/data
download_dataset.py __MACOSX source.txt UCI HAR Dataset UCI HAR Dataset.zip
/home/ubuntu/pynb/LSTM-Human-Activity-Recognition
data LSTM_files LSTM_OLD.ipynb README.md
LICENSE LSTM.ipynb lstm.py screenlog.0
Dataset is now located at: data/UCI HAR Dataset/
## Preparing dataset:
```python
TRAIN = "train/"
TEST = "test/"
# Load "X" (the neural network's training and testing inputs)
def load_X(X_signals_paths):
X_signals = []
for signal_type_path in X_signals_paths:
file = open(signal_type_path, 'r')
# Read dataset from disk, dealing with text files' syntax
X_signals.append(
[np.array(serie, dtype=np.float32) for serie in [
row.replace(' ', ' ').strip().split(' ') for row in file
]]
)
file.close()
return np.transpose(np.array(X_signals), (1, 2, 0))
X_train_signals_paths = [
DATASET_PATH + TRAIN + "Inertial Signals/" + signal + "train.txt" for signal in INPUT_SIGNAL_TYPES
]
X_test_signals_paths = [
DATASET_PATH + TEST + "Inertial Signals/" + signal + "test.txt" for signal in INPUT_SIGNAL_TYPES
]
X_train = load_X(X_train_signals_paths)
X_test = load_X(X_test_signals_paths)
# Load "y" (the neural network's training and testing outputs)
def load_y(y_path):
file = open(y_path, 'r')
# Read dataset from disk, dealing with text file's syntax
y_ = np.array(
[elem for elem in [
row.replace(' ', ' ').strip().split(' ') for row in file
]],
dtype=np.int32
)
file.close()
# Substract 1 to each output class for friendly 0-based indexing
return y_ - 1
y_train_path = DATASET_PATH + TRAIN + "y_train.txt"
y_test_path = DATASET_PATH + TEST + "y_test.txt"
y_train = load_y(y_train_path)
y_test = load_y(y_test_path)
```
## Additionnal Parameters:
Here are some core parameter definitions for the training.
The whole neural network's structure could be summarised by enumerating those parameters and the fact an LSTM is used.
```python
# Input Data
training_data_count = len(X_train) # 7352 training series (with 50% overlap between each serie)
test_data_count = len(X_test) # 2947 testing series
n_steps = len(X_train[0]) # 128 timesteps per series
n_input = len(X_train[0][0]) # 9 input parameters per timestep
# LSTM Neural Network's internal structure
n_hidden = 32 # Hidden layer num of features
n_classes = 6 # Total classes (should go up, or should go down)
# Training
learning_rate = 0.0025
lambda_loss_amount = 0.0015
training_iters = training_data_count * 300 # Loop 300 times on the dataset
batch_size = 1500
display_iter = 30000 # To show test set accuracy during training
# Some debugging info
print("Some useful info to get an insight on dataset's shape and normalisation:")
print("(X shape, y shape, every X's mean, every X's standard deviation)")
print(X_test.shape, y_test.shape, np.mean(X_test), np.std(X_test))
print("The dataset is therefore properly normalised, as expected, but not yet one-hot encoded.")
```
Some useful info to get an insight on dataset's shape and normalisation:
(X shape, y shape, every X's mean, every X's standard deviation)
(2947, 128, 9) (2947, 1) 0.0991399 0.395671
The dataset is therefore properly normalised, as expected, but not yet one-hot encoded.
## Utility functions for training:
```python
def LSTM_RNN(_X, _weights, _biases):
# Function returns a tensorflow LSTM (RNN) artificial neural network from given parameters.
# Moreover, two LSTM cells are stacked which adds deepness to the neural network.
# Note, some code of this notebook is inspired from an slightly different
# RNN architecture used on another dataset, some of the credits goes to
# "aymericdamien" under the MIT license.
# (NOTE: This step could be greatly optimised by shaping the dataset once
# input shape: (batch_size, n_steps, n_input)
_X = tf.transpose(_X, [1, 0, 2]) # permute n_steps and batch_size
# Reshape to prepare input to hidden activation
_X = tf.reshape(_X, [-1, n_input])
# new shape: (n_steps*batch_size, n_input)
# Linear activation
_X = tf.nn.relu(tf.matmul(_X, _weights['hidden']) + _biases['hidden'])
# Split data because rnn cell needs a list of inputs for the RNN inner loop
_X = tf.split(_X, n_steps, 0)
# new shape: n_steps * (batch_size, n_hidden)
# Define two stacked LSTM cells (two recurrent layers deep) with tensorflow
lstm_cell_1 = tf.contrib.rnn.BasicLSTMCell(n_hidden, forget_bias=1.0, state_is_tuple=True)
lstm_cell_2 = tf.contrib.rnn.BasicLSTMCell(n_hidden, forget_bias=1.0, state_is_tuple=True)
lstm_cells = tf.contrib.rnn.MultiRNNCell([lstm_cell_1, lstm_cell_2], state_is_tuple=True)
# Get LSTM cell output
outputs, states = tf.contrib.rnn.static_rnn(lstm_cells, _X, dtype=tf.float32)
# Get last time step's output feature for a "many to one" style classifier,
# as in the image describing RNNs at the top of this page
lstm_last_output = outputs[-1]
# Linear activation
return tf.matmul(lstm_last_output, _weights['out']) + _biases['out']
def extract_batch_size(_train, step, batch_size):
# Function to fetch a "batch_size" amount of data from "(X|y)_train" data.
shape = list(_train.shape)
shape[0] = batch_size
batch_s = np.empty(shape)
for i in range(batch_size):
# Loop index
index = ((step-1)*batch_size + i) % len(_train)
batch_s[i] = _train[index]
return batch_s
def one_hot(y_):
# Function to encode output labels from number indexes
# e.g.: [[5], [0], [3]] --> [[0, 0, 0, 0, 0, 1], [1, 0, 0, 0, 0, 0], [0, 0, 0, 1, 0, 0]]
y_ = y_.reshape(len(y_))
n_values = int(np.max(y_)) + 1
return np.eye(n_values)[np.array(y_, dtype=np.int32)] # Returns FLOATS
```
## Let's get serious and build the neural network:
```python
# Graph input/output
x = tf.placeholder(tf.float32, [None, n_steps, n_input])
y = tf.placeholder(tf.float32, [None, n_classes])
# Graph weights
weights = {
'hidden': tf.Variable(tf.random_normal([n_input, n_hidden])), # Hidden layer weights
'out': tf.Variable(tf.random_normal([n_hidden, n_classes], mean=1.0))
}
biases = {
'hidden': tf.Variable(tf.random_normal([n_hidden])),
'out': tf.Variable(tf.random_normal([n_classes]))
}
pred = LSTM_RNN(x, weights, biases)
# Loss, optimizer and evaluation
l2 = lambda_loss_amount * sum(
tf.nn.l2_loss(tf_var) for tf_var in tf.trainable_variables()
) # L2 loss prevents this overkill neural network to overfit the data
cost = tf.reduce_mean(tf.nn.softmax_cross_entropy_with_logits(labels=y, logits=pred)) + l2 # Softmax loss
optimizer = tf.train.AdamOptimizer(learning_rate=learning_rate).minimize(cost) # Adam Optimizer
correct_pred = tf.equal(tf.argmax(pred,1), tf.argmax(y,1))
accuracy = tf.reduce_mean(tf.cast(correct_pred, tf.float32))
```
## Hooray, now train the neural network:
```python
# To keep track of training's performance
test_losses = []
test_accuracies = []
train_losses = []
train_accuracies = []
# Launch the graph
sess = tf.InteractiveSession(config=tf.ConfigProto(log_device_placement=True))
init = tf.global_variables_initializer()
sess.run(init)
# Perform Training steps with "batch_size" amount of example data at each loop
step = 1
while step * batch_size <= training_iters:
batch_xs = extract_batch_size(X_train, step, batch_size)
batch_ys = one_hot(extract_batch_size(y_train, step, batch_size))
# Fit training using batch data
_, loss, acc = sess.run(
[optimizer, cost, accuracy],
feed_dict={
x: batch_xs,
y: batch_ys
}
)
train_losses.append(loss)
train_accuracies.append(acc)
# Evaluate network only at some steps for faster training:
if (step*batch_size % display_iter == 0) or (step == 1) or (step * batch_size > training_iters):
# To not spam console, show training accuracy/loss in this "if"
print("Training iter #" + str(step*batch_size) + \
": Batch Loss = " + "{:.6f}".format(loss) + \
", Accuracy = {}".format(acc))
# Evaluation on the test set (no learning made here - just evaluation for diagnosis)
loss, acc = sess.run(
[cost, accuracy],
feed_dict={
x: X_test,
y: one_hot(y_test)
}
)
test_losses.append(loss)
test_accuracies.append(acc)
print("PERFORMANCE ON TEST SET: " + \
"Batch Loss = {}".format(loss) + \
", Accuracy = {}".format(acc))
step += 1
print("Optimization Finished!")
# Accuracy for test data
one_hot_predictions, accuracy, final_loss = sess.run(
[pred, accuracy, cost],
feed_dict={
x: X_test,
y: one_hot(y_test)
}
)
test_losses.append(final_loss)
test_accuracies.append(accuracy)
print("FINAL RESULT: " + \
"Batch Loss = {}".format(final_loss) + \
", Accuracy = {}".format(accuracy))
```
WARNING:tensorflow:From
:9: initialize_all_variables (from tensorflow.python.ops.variables) is deprecated and will be removed after 2017-03-02.
Instructions for updating:
Use `tf.global_variables_initializer` instead.
Training iter #1500: Batch Loss = 5.416760, Accuracy = 0.15266665816307068
PERFORMANCE ON TEST SET: Batch Loss = 4.88082***11096191, Accuracy = 0.056328471750020***
Training iter #30000: Batch Loss = 3.031930, Accuracy = 0.607333242893219
PERFORMANCE ON TEST SET: Batch Loss = 3.0515167713165283, Accuracy = 0.6067186594009399
Training iter #60000: Batch Loss = 2.6727***, Accuracy = 0.7386666536331177
PERFORMANCE ON TEST SET: Batch Loss = 2.780435085296631, Accuracy = 0.7027485370635***6
Training iter #90000: Batch Loss = 2.378301, Accuracy = 0.8366667032241821
PERFORMANCE ON TEST SET: Batch Loss = 2.6019773483276367, Accuracy = 0.7617915868759155
Training iter #120000: Batch Loss = 2.127290, Accuracy = 0.9066667556762695
PERFORMANCE ON TEST SET: Batch Loss = 2.3625404834747314, Accuracy = 0.8116728663444519
Training iter #150000: Batch Loss = 1.92***05, Accuracy = 0.9380000233650208
PERFORMANCE ON TEST SET: Batch Loss = 2.306251049041748, Accuracy = 0.8276212215423584
Training iter #180000: Batch Loss = 1.971904, Accuracy = 0.9153333902359009
PERFORMANCE ON TEST SET: Batch Loss = 2.0835530757904053, Accuracy = 0.8771631121635437
Training iter #210000: Batch Loss = 1.860249, Accuracy = 0.8613333702087402
PERFORMANCE ON TEST SET: Batch Loss = 1.9994492530822754, Accuracy = 0.8788597583770752
Training iter #240000: Batch Loss = 1.626292, Accuracy = 0.9380000233650208
PERFORMANCE ON TEST SET: Batch Loss = 1.879166603088379, Accuracy = 0.8944689035415***9
Training iter #270000: Batch Loss = 1.582758, Accuracy = 0.9386667013168335
PERFORMANCE ON TEST SET: Batch Loss = 2.0341007709503174, Accuracy = 0.8361043930053711
Training iter #300000: Batch Loss = 1.620352, Accuracy = 0.9306666851043701
PERFORMANCE ON TEST SET: Batch Loss = 1.8185184001922607, Accuracy = 0.863929331302***28
Training iter #330000: Batch Loss = 1.474394, Accuracy = 0.9693333506584167
PERFORMANCE ON TEST SET: Batch Loss = 1.76385033130***575, Accuracy = 0.8747878670692444
Training iter #360000: Batch Loss = 1.4069***, Accuracy = 0.9420000314712524
PERFORMANCE ON TEST SET: Batch Loss = 1.5946787595748901, Accuracy = 0.902273416519165
Training iter #390000: Batch Loss = 1.362515, Accuracy = 0.940000057220459
PERFORMANCE ON TEST SET: Batch Loss = 1.5285792350769043, Accuracy = 0.904***87212181091
Training iter #420000: Batch Loss = 1.252860, Accuracy = 0.9566667079925537
PERFORMANCE ON TEST SET: Batch Loss = 1.4635565280914307, Accuracy = 0.910756587***21777
Training iter #450000: Batch Loss = 1.190078, Accuracy = 0.9553333520889282
PERFORMANCE ON TEST SET: Batch Loss = 1.442753553390503, Accuracy = 0.9093992710113525
Training iter #480000: Batch Loss = 1.159610, Accuracy = 0.9446667432785034
PERFORMANCE ON TEST SET: Batch Loss = 1.4130011796951294, Accuracy = 0.8971834778785706
Training iter #510000: Batch Loss = 1.100551, Accuracy = 0.9593333601951599
PERFORMANCE ON TEST SET: Batch Loss = 1.3075592517852783, Accuracy = 0.9117745757102966
Training iter #540000: Batch Loss = 1.123470, Accuracy = 0.9240000247955322
PERFORMANCE ON TEST SET: Batch Loss = 1.2605488300323486, Accuracy = 0.9165251851081848
Training iter #570000: Batch Loss = 1.103454, Accuracy = 0.909333348274231
PERFORMANCE ON TEST SET: Batch Loss = 1.2327136993408203, Accuracy = 0.9009160399436951
Training iter #600000: Batch Loss = 1.083368, Accuracy = 0.896666***60037231
PERFORMANCE ON TEST SET: Batch Loss = 1.2683708667755127, Accuracy = 0.88903951***489746
Training iter #630000: Batch Loss = 0.939185, Accuracy = 0.9700000882148743
PERFORMANCE ON TEST SET: Batch Loss = 1.2147629261016846, Accuracy = 0.8866***2713546753
Training iter #660000: Batch Loss = 0.881242, Accuracy = 0.***06667566299438
PERFORMANCE ON TEST SET: Batch Loss = 1.1068334579467773, Accuracy = 0.9151678681373596
Training iter #690000: Batch Loss = 0.831674, Accuracy = 0.***5333442687***83
PERFORMANCE ON TEST SET: Batch Loss = 1.0885852575302124, Accuracy = 0.9121139***557***77
Training iter #720000: Batch Loss = 0.866615, Accuracy = 0.9573334455490112
PERFORMANCE ON TEST SET: Batch Loss = 1.05135166***505005, Accuracy = 0.9158465266227722
Training iter #750000: Batch Loss = 0.858979, Accuracy = 0.940000057220459
PERFORMANCE ON TEST SET: Batch Loss = 1.05***633289337158, Accuracy = 0.906345367431***06
Training iter #780000: Batch Loss = 0.750040, Accuracy = 0.95933341979***047
PERFORMANCE ON TEST SET: Batch Loss = 1.01096***20173***5, Accuracy = 0.9155071973800659
Training iter #810000: Batch Loss = 0.732136, Accuracy = 0.9620000123977661
PERFORMANCE ON TEST SET: Batch Loss = 0.***6569***30206299, Accuracy = 0.9161 ... ...